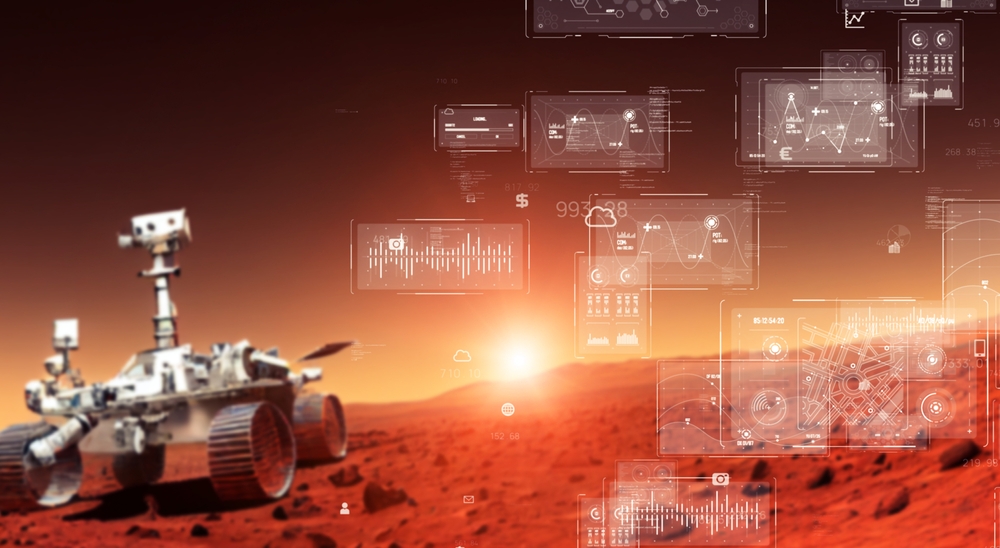
Scientists at MIT and the University of Basel have developed a new AI technique that improves how researchers study phase transitions in materials. Traditional methods for detecting these changes, like when water turns to ice, require deep theoretical knowledge and tend to be labor-intensive. The new method utilizes generative AI to automatically map out phase diagrams for unknown physical systems efficiently, without the need for large, labeled datasets.
This approach is particularly useful for examining novel materials and could potentially reveal new phases of matter autonomously. By leveraging the generative models, the AI can classify phases and transitions based on a system’s inherent properties. This allows for faster and potentially more accurate scientific discoveries, as it reduces human bias and the need for extensive data training.
Frank Schäfer from MIT’s Julia Lab, along with researchers Julian Arnold, Alan Edelman, and Christoph Bruder, demonstrated this technique’s effectiveness. Their work, Mapping Out Phase Diagrams with Generative Classifiers published in Physical Review Letters, shows that generative AI can identify phase changes by directly constructing classifiers from physical system simulations, which are already informed by existing scientific techniques.
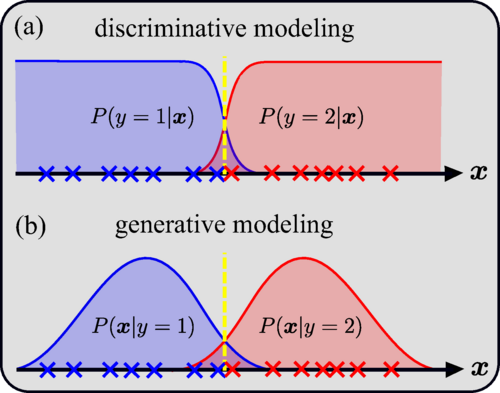
This streamlined method promises to expedite the detection of phase transitions, enhance computational efficiency, and could even be adapted to solve various classification problems in physics, such as detecting entanglement in quantum systems or optimizing parameters in large language models like ChatGPT. The team is now exploring the theoretical limits of this technique, including how few measurements are necessary to effectively detect phase changes.
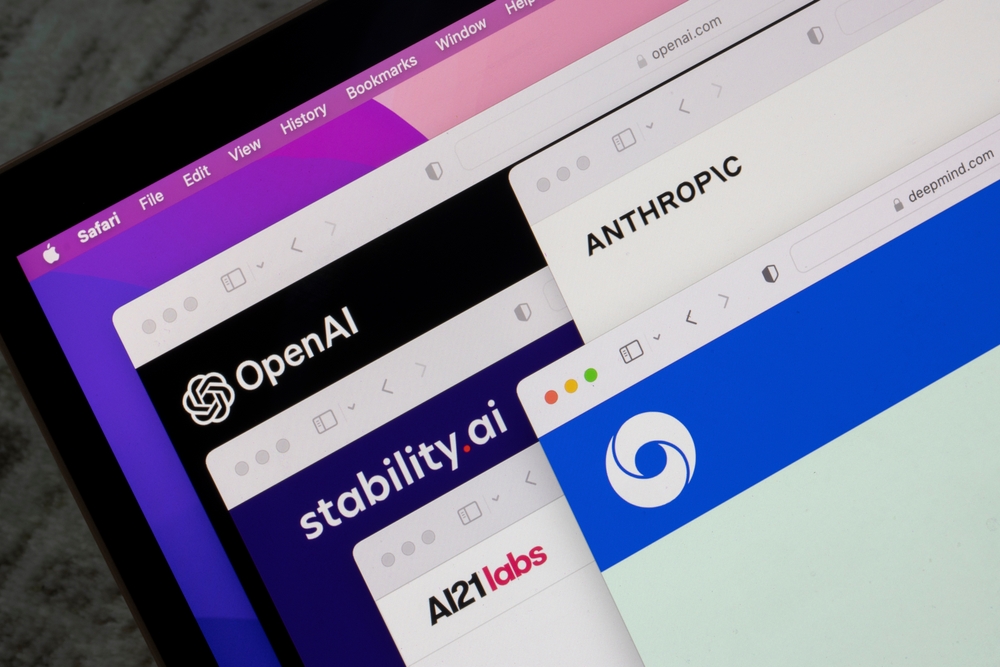